Copyright © 2024
Adaptive ML, Inc.
All rights reserved
Adaptive ML, Inc.
All rights reserved
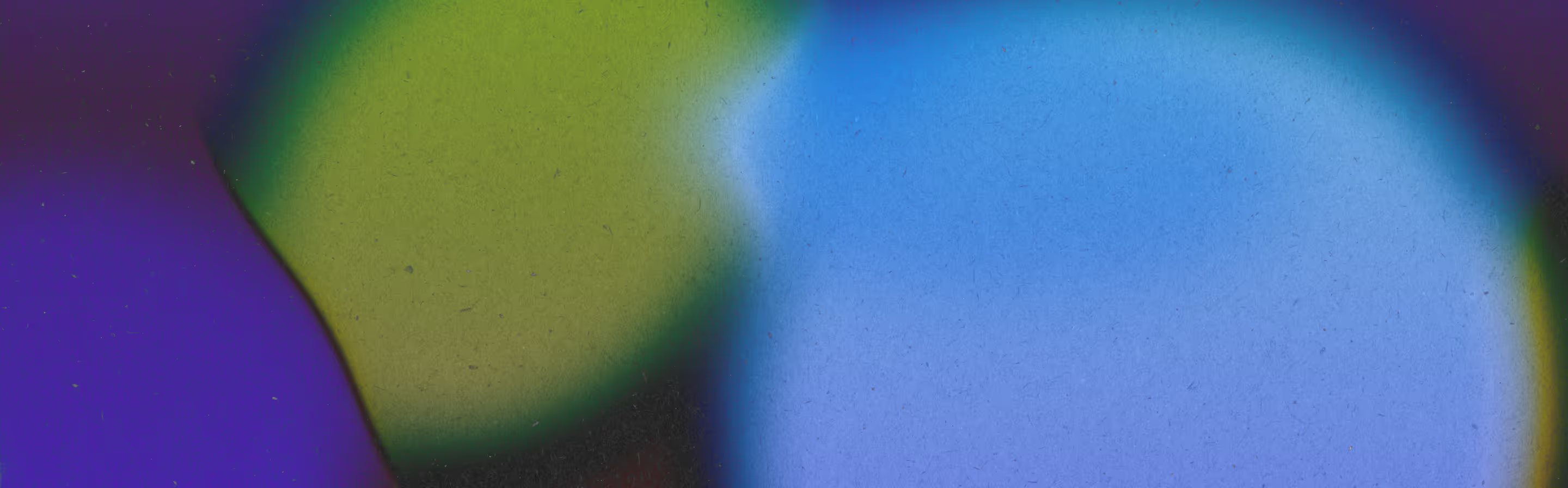
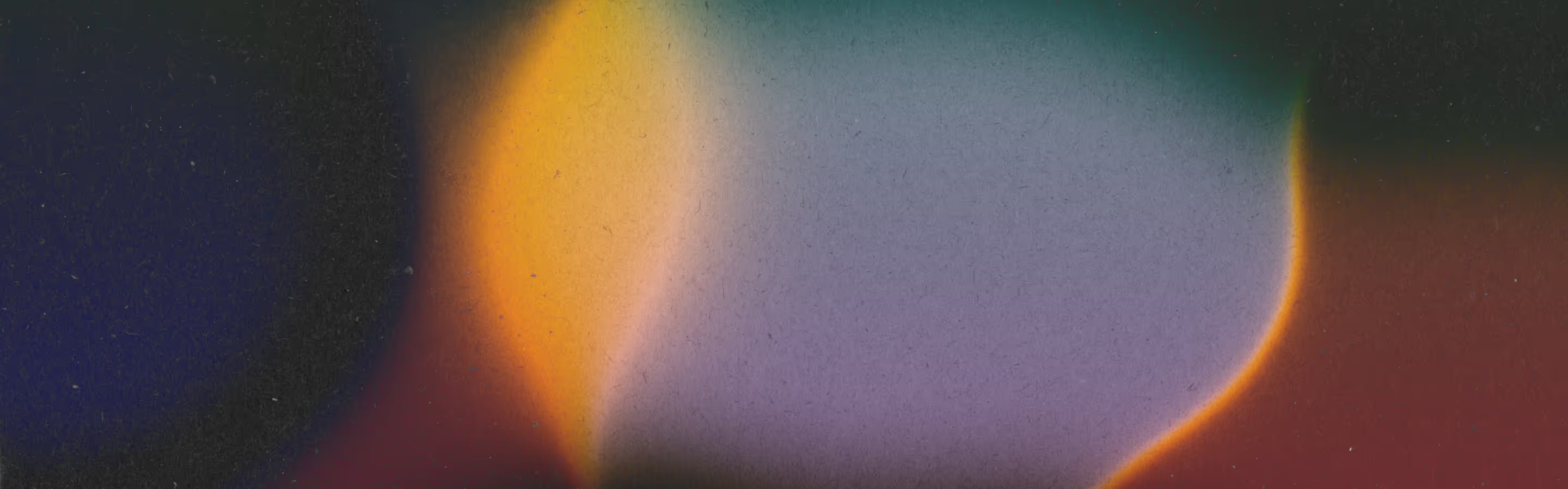

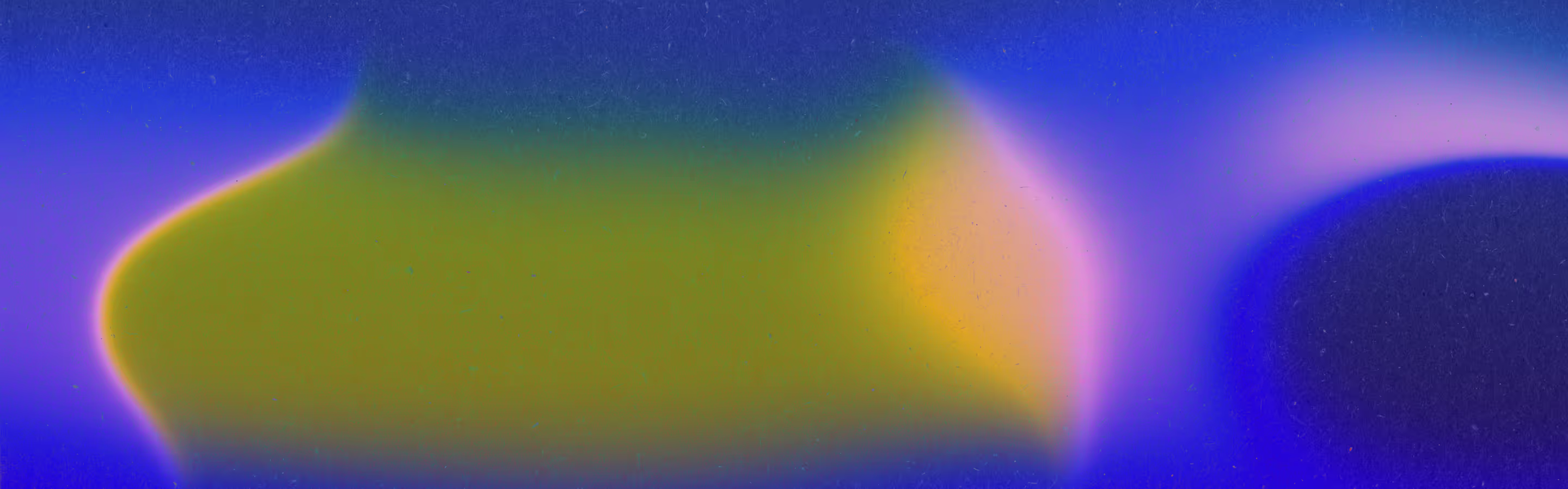
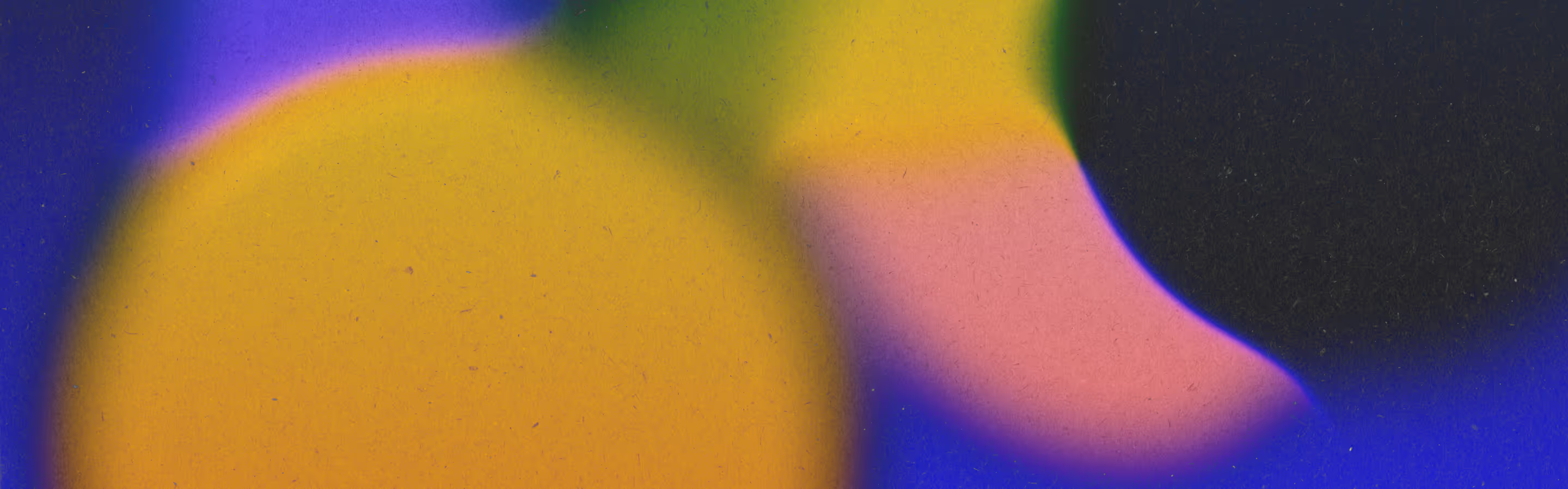
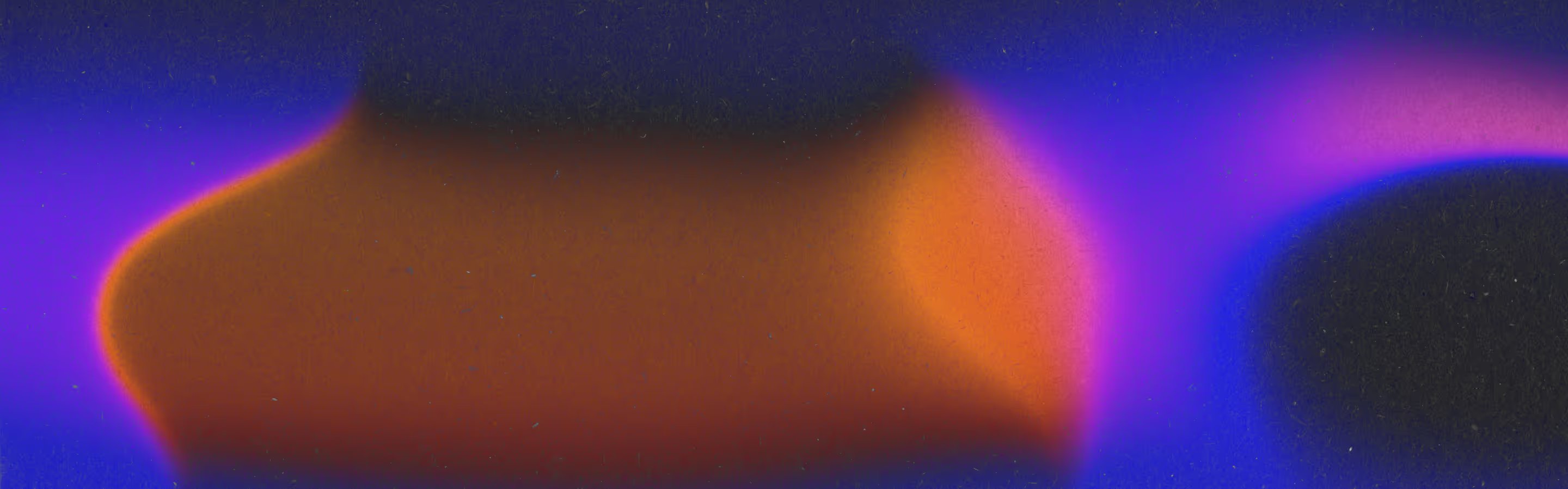
‘Scaling Laws’ and Reasoning Dominate NeurIPS
Whether or not this “scaling wall” hypothesis is true has huge implications on both industry and academia. Few people believe that progress will fully stop, but if AI labs shift to spending more computational resources at the time of inference (or, when users ask AI models questions) rather than during the model training process, AI developers with fewer resources and compute may be more easily able to catch up, as we discussed in this story. That could even the playing field for smaller AI startups and academic labs.
The idea that progress from traditional scaling could be slowing has support from some of the biggest names in the AI industry, like Ilya Sutskever, the former chief scientist at OpenAI who earlier this year cofounded his own competing AI lab.
During a NeurIPS keynote, Sutskever said that "pretraining will end" because while "compute is growing through better hardware, better algorithms and larger clusters... data is not growing because we have but one internet."
So it’s not surprising that many conversations at NeurIPS centered around the so-called “reasoning” models that OpenAI has popularized, and theories about how the ChatGPT maker was able to train them. Many of those theories involve reinforcement learning, a model training method that’s based on rewarding desired behaviors and punishing undesired ones, which was another popular topic of conversation at NeurIPS, said Julien Launay, cofounder and CEO at Adaptive ML, a startup that helps developers customize open-source models.