
Enterprise
Search
Reduce model

Challenge
In production, model hallucinations are unacceptable.
To help models stick to the facts, enterprises provide models with relevant documents to answer from using a Retrieval Augmented Generation (RAG) system.
However, these systems do not guarantee correct use of the provided documents: models can hallucinate, answering users with unsupported information.

Solution
Models tuned to your use case and business documents.
Adaptive Engine allows organizations to tune specialized models that can reduce hallucinations and improve user satisfaction.
By generating synthetic data directly from your documents, Adaptive Engine eliminates the need for data collection, delivering a RAG-optimized model on day one.

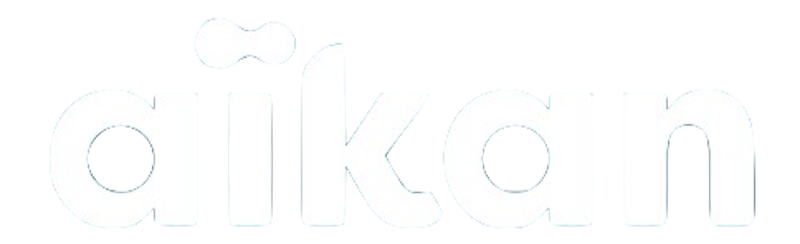
Delivering faithful RAG on legal documents
Aïkan is a French LegalTech company building AI-powered software for insurance companies. Aïkan is working with Adaptive ML to develop steerable, specialized models that are highly faithful to their RAG context.
Proprietary models struggle to follow RAG guidelines, occasionally hallucinating incorrect or unsupported information.
Using a combination of production and synthetic data, as well as feedback from expert annotators, Adaptive ML tuned a small, specialized model to be more faithful and accurate than GPT-4o.
The tuned model cites its sources, and presents its findings in a precise format preferred by legal clerks.
EFFECTIVE
Adaptive ML tuned a Llama 3.1 8B model, reducing hallucinations by 25% over GPT-4o, and 42% over Llama 3.1 8B Instruct.
EFFICIENT
Adaptive ML models were trained using synthetic annotations, significantly reducing production time and costs.
EXTENSIBLE
The proposed solution is extensible; models continuously improve over time, learning from user interactions.

Workflow
Speed to production
Tune models quickly using synthetic data generated from your document corpus.
Improve RAG accuracy
Minimize model hallucinations using an AI judge to give feedback on faithfulness.
Smaller models, smaller cost
Use models as small as 8B to outperform frontier models, while reducing cost.